As the world becomes increasingly connected, the need for faster, more reliable data processing has never been more critical. Traditional cloud computing, while powerful, often struggles to meet the demands of real-time applications that require immediate data analysis and response.
This is where edge computing comes in—a decentralized computing model that processes data closer to its source, minimizing latency and enhancing speed. By bringing data processing to the “edge” of the network, closer to devices like sensors, cameras, or local servers, edge computing is revolutionizing industries ranging from manufacturing to healthcare.
In parallel, the rise of real-time data testing is transforming how systems are monitored and maintained. Real-time data testing ensures that systems are capable of handling live data streams with precision, accuracy, and efficiency. It plays a pivotal role in identifying issues or bottlenecks before they impact system performance, ensuring seamless operation in time-sensitive environments.
Together, edge computing and real-time data testing form a powerful combination that significantly enhances the reliability and performance of complex systems. Whether it’s an autonomous vehicle reacting to changing road conditions, a factory using IoT devices for predictive maintenance, or a smart city optimizing traffic flow, the convergence of these technologies ensures that systems remain robust, efficient, and responsive.
In this article, we will explore how edge computing, coupled with real-time data testing, is shaping the future of technology and providing new avenues for improving system reliability across a wide range of applications.
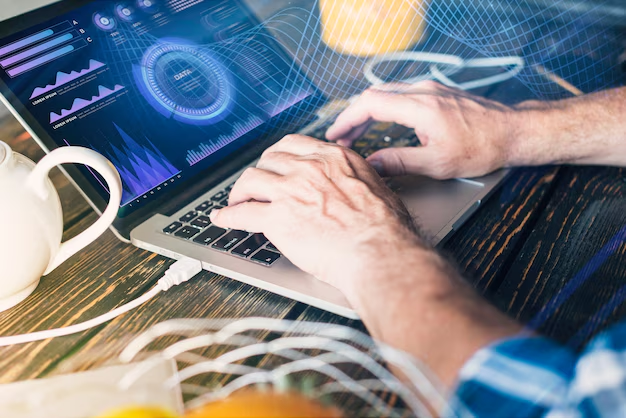
Role of Real-Time Data Testing in Edge Computing
In the context of edge computing, where data is processed closer to its source, the role of real-time data testing becomes essential for ensuring that systems operate with accuracy, speed, and reliability. Edge environments often handle time-sensitive data, meaning that delays, inaccuracies, or malfunctions can have critical consequences, especially in applications like autonomous vehicles, industrial IoT, or healthcare monitoring. Real-time data testing helps mitigate these risks by continuously validating the integrity, performance, and functionality of edge devices and systems under live conditions.
1. Ensuring Data Integrity and Consistency
One of the core functions of real-time data testing is to ensure that the data being processed at the edge is accurate and reliable. In decentralized systems, edge devices such as sensors, cameras, and smart gateways collect vast amounts of data. Real-time testing ensures that this data is properly formatted, transmitted, and synchronized across distributed networks. It identifies any inconsistencies or corruptions in data flow, which, if left unchecked, could lead to incorrect decisions or faulty operations in edge-based applications.
2. Addressing Time Sensitivity
Many edge computing applications, such as autonomous vehicles or smart manufacturing systems, require immediate processing and response. There is little room for delay or error, as latency in data handling can result in safety risks or operational inefficiencies. Real-time data testing verifies that systems can meet the time-sensitive demands of edge environments, ensuring that data is processed and acted upon within milliseconds. For example, in an autonomous car, real-time testing validates that the vehicle’s sensors and AI algorithms can accurately detect obstacles and react quickly, avoiding potential accidents.
3. Detecting and Preventing System Failures
System reliability is a primary concern in edge computing, as edge devices operate independently of centralized data centers. Real-time data testing plays a vital role in detecting potential system failures before they occur. By continuously monitoring the performance of edge devices, real-time testing can identify early warning signs of hardware malfunctions, software bugs, or network interruptions. This proactive approach ensures that issues are addressed promptly, minimizing downtime and ensuring the uninterrupted operation of mission-critical systems.
4. Enhancing Security and Compliance
Security is a significant concern in edge environments, where data is often processed outside the secure confines of traditional data centers. Real-time data testing helps bolster security by continuously evaluating the flow of data for any vulnerabilities or breaches. For example, real-time testing can identify abnormal data patterns that may indicate a cyberattack or unauthorized access to an edge device. By integrating real-time testing with security protocols, organizations can quickly detect and respond to potential threats, safeguarding sensitive data and ensuring compliance with industry standards.
5. Optimizing Performance in Distributed Systems
In edge computing, multiple devices often work in tandem across a distributed network. Coordinating and maintaining the performance of these devices is critical for smooth operation. Real-time data testing ensures that each component in the edge network is functioning optimally. This includes validating the efficiency of communication between devices, assessing the speed and accuracy of data processing, and identifying any bottlenecks that may hinder performance. Through continuous testing, organizations can optimize the performance of their edge systems, improving both speed and reliability.
6. Testing in Dynamic and Harsh Environments
Many edge computing applications operate in dynamic or harsh environments, such as offshore oil rigs, remote industrial sites, or aerospace systems. These environments present unique challenges, such as fluctuating network connectivity, extreme temperatures, and limited access to centralized resources. Real-time data testing helps overcome these challenges by ensuring that edge systems can adapt to changing conditions. It validates the resilience of edge devices, ensuring that they continue to function even when network conditions are unstable or environmental factors are extreme.
7. Supporting Continuous Data Flow and Processing
Edge computing requires constant data flow and processing to achieve the low-latency, high-performance benefits it promises. Real-time data testing supports this by continuously verifying that data flows uninterrupted from edge devices to local processing units. This real-time monitoring ensures that any interruptions, delays, or inconsistencies in data transmission are immediately identified and addressed, enabling edge systems to maintain a continuous flow of real-time data.
Enhancing System Reliability through Edge Computing
Edge computing is revolutionizing the way modern systems process data by shifting computation closer to where it is generated, rather than relying solely on distant centralized servers. This approach is not only reducing latency and increasing data processing speeds, but it is also significantly enhancing system reliability across a variety of industries. As more applications require real-time decision-making and data handling—whether in autonomous vehicles, industrial automation, or healthcare—ensuring the reliability of these systems becomes crucial for operational success.
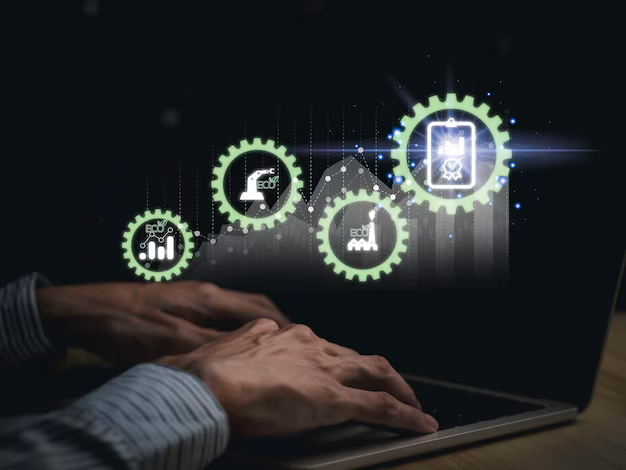
1. Faster Response Times and Reduced Latency
One of the primary advantages of edge computing is the ability to process data closer to its source, which drastically reduces latency. In traditional cloud-based systems, data often has to travel over long distances to centralized data centers for processing, resulting in delays that can be unacceptable for time-sensitive applications. Edge computing, by contrast, ensures that data is processed locally, cutting down the time it takes to generate actionable insights or trigger automatic responses.
For instance, in autonomous vehicles, immediate decision-making is critical to avoid accidents. Any delay in processing sensor data—whether from cameras, radar, or LiDAR—could have catastrophic consequences. By using edge computing, real-time data is processed within milliseconds, enabling split-second decisions. This ability to act immediately enhances the reliability of the overall system by ensuring consistent and predictable behavior even under high-stress conditions.
2. Decentralized Processing Reduces Single Points of Failure
Centralized systems often face the risk of a single point of failure, where an issue at a central server can disrupt the entire network. Edge computing addresses this vulnerability by distributing data processing across multiple edge nodes, making the system more resilient to failures. If one edge node goes offline or malfunctions, other nodes can continue to operate without disrupting the entire system.
In industrial automation, for example, smart machines and robots depend on uninterrupted data streams to maintain production efficiency. If data processing were solely dependent on a centralized cloud, any server failure could lead to costly downtime. By distributing the processing across edge devices within the factory, system reliability is greatly improved as the production line continues to operate without interruption, even if one or more devices encounter issues.
3. Improved Fault Tolerance and Redundancy
Another way edge computing enhances reliability is by improving fault tolerance. Edge devices can store and process data locally, allowing them to continue operating independently, even when disconnected from the central cloud. This redundancy is especially valuable in environments where connectivity can be inconsistent, such as in remote or rural locations, or in industries like oil and gas or mining, where systems are often deployed in isolated areas.
For instance, in healthcare applications, edge computing can ensure uninterrupted data processing for critical patient monitoring systems in remote hospitals. Even if the network connection to a cloud server is temporarily lost, local edge devices can continue to process and store data, alerting healthcare providers to any abnormalities in real-time. This increases the reliability of these systems, safeguarding against potential connectivity failures.
4. Enhanced Data Security
Security is a significant concern in today’s hyperconnected world, especially for applications dealing with sensitive or confidential data, such as healthcare, finance, and defense. Edge computing enhances security by localizing data processing, meaning that sensitive data doesn’t have to be transmitted across the internet to distant servers for analysis. This reduces the risk of data breaches during transmission and ensures that critical information is protected.
In sectors like defense and aerospace, where data sensitivity is paramount, processing data locally on edge devices ensures that it remains secure and reduces the chances of external attacks. Even in the case of a cyberattack targeting the central system, the localized edge nodes are better isolated and can continue functioning securely without compromising the system’s integrity.
5. Real-Time Monitoring and Self-Correction
Edge computing enables continuous real-time monitoring of systems, which in turn enhances reliability by allowing for proactive detection of faults and anomalies. Many edge systems are equipped with built-in monitoring tools that can automatically identify and respond to potential issues. These self-correcting systems can address minor faults or performance drops before they become critical, reducing the likelihood of system failure.
For example, in smart grids, edge computing allows for real-time monitoring of electricity usage and supply. If a fault is detected, such as a power overload or equipment failure, the edge system can instantly correct the issue or reroute power flows to prevent outages. This real-time adaptability ensures greater system reliability by preventing small issues from escalating into larger problems.
6. Scalability and Flexibility
As systems expand and grow more complex, maintaining reliability becomes more challenging. Edge computing offers the scalability and flexibility needed to accommodate this growth without sacrificing system reliability. By decentralizing data processing and enabling each edge device to handle its own data independently, edge computing supports the seamless integration of new devices and data sources without overwhelming the system.
This is particularly relevant in smart city applications, where thousands of sensors and IoT devices must communicate and operate efficiently. Edge computing ensures that the system remains scalable while maintaining consistent reliability, even as the number of devices and data streams increases.
Tools and Technologies for Real-Time Data Testing
As the demand for real-time data testing in edge computing and other environments grows, a variety of tools and technologies have emerged to meet this need. These tools enable organizations to ensure the integrity, performance, and reliability of systems that process live data. Below are some of the most effective tools and technologies utilized in real-time data testing, along with their key features and applications.
1. Apache Kafka
Apache Kafka is a distributed streaming platform widely used for building real-time data pipelines and streaming applications. It allows for high-throughput, fault-tolerant data streaming, making it an excellent choice for real-time data testing.
- Key Features:
- Pub/Sub messaging model for decoupling data producers and consumers.
- Scalability to handle large volumes of data across multiple nodes.
- Support for real-time analytics through stream processing capabilities.
- Applications: Ideal for monitoring and testing applications that require continuous data feeds, such as financial services, IoT sensor data, and social media platforms.
2. Apache Flink
Apache Flink is a powerful stream processing framework designed for real-time data analytics. It excels in handling complex event processing and is particularly useful for applications requiring low-latency data processing.
- Key Features:
- Event time processing for accurate handling of time-sensitive data.
- Built-in support for stateful computations, allowing for dynamic data handling.
- Fault tolerance and exactly-once processing semantics.
- Applications: Used in scenarios such as fraud detection, real-time recommendations, and monitoring for industrial IoT systems.
3. TensorFlow Extended (TFX)
TensorFlow Extended is an end-to-end platform designed for deploying production machine learning pipelines. It incorporates real-time testing capabilities to ensure that models are functioning correctly in live environments.
- Key Features:
- Components for data validation, model evaluation, and serving.
- Support for monitoring model performance and data drift in real time.
- Integration with various data sources for real-time inference.
- Applications: Suitable for organizations deploying machine learning models in real-time applications, such as recommendation systems, predictive maintenance, and healthcare diagnostics.
4. Prometheus
Prometheus is an open-source monitoring and alerting toolkit that is particularly well-suited for real-time testing of systems and services. It collects metrics from configured targets at specified intervals and provides powerful querying capabilities.
- Key Features:
- Multi-dimensional data model with time series data.
- Built-in alerting capabilities based on user-defined thresholds.
- Easy integration with cloud-native environments and microservices.
- Applications: Often used in conjunction with containerized applications and microservices architectures to monitor performance and detect anomalies in real time.
5. Grafana
Grafana is an open-source platform for data visualization and monitoring. It works well with various data sources, including Prometheus, and allows users to create dashboards for real-time monitoring of systems.
- Key Features:
- Customizable dashboards for visualizing metrics and logs.
- Alerts based on specific conditions to ensure timely responses.
- Support for multiple data sources and plugins for enhanced functionality.
- Applications: Used for visualizing real-time performance metrics, operational analytics, and monitoring complex distributed systems.
6. JMeter
Apache JMeter is a popular open-source tool for performance testing, primarily used for web applications. It can also be adapted for real-time data testing scenarios to assess the performance of various services under load.
- Key Features:
- Ability to simulate heavy loads on servers to evaluate performance.
- Support for multiple protocols, including HTTP, FTP, and JMS.
- Real-time monitoring of test results and system performance.
- Applications: Useful for load testing APIs, web applications, and services that require real-time data processing.
7. Katalon Studio
Katalon Studio is an integrated testing solution that supports web, mobile, and API testing. It can be employed for real-time testing of applications by integrating with various monitoring tools and frameworks.
- Key Features:
- Comprehensive test management capabilities.
- Support for both manual and automated testing.
- Real-time reporting and analytics.
- Applications: Suitable for testing applications that require constant monitoring and validation of live data, particularly in web and mobile contexts.
8. Nagios
Nagios is an open-source monitoring system that provides monitoring and alerting services for servers, networks, and applications. It plays a crucial role in real-time data testing by ensuring system availability and performance.
- Key Features:
- Comprehensive monitoring of network services and server resources.
- Notification system for alerting on potential issues in real time.
- Customizable plugins for tailored monitoring solutions.
- Applications: Used in IT infrastructure monitoring, ensuring that critical systems and applications remain operational and efficient.
9. DataRobot
DataRobot is an automated machine-learning platform that simplifies the development and deployment of machine-learning models. It includes real-time monitoring and testing capabilities to ensure model performance remains optimal in live environments.
- Key Features:
- Automated model evaluation and monitoring.
- Integration with various data sources for real-time analytics.
- Continuous learning capabilities to adapt to changing data patterns.
- Applications: Ideal for organizations leveraging machine learning for real-time insights in sectors like finance, marketing, and healthcare.
Conclusion
In an era where data is generated at an unprecedented rate, the importance of real-time data testing cannot be overstated. As organizations increasingly adopt edge computing architectures to enhance responsiveness and efficiency, real-time data testing becomes a critical component in ensuring the reliability, security, and performance of systems. The integration of robust tools and technologies, such as Apache Kafka, Prometheus, and TensorFlow Extended, empowers businesses to monitor, validate, and optimize their data processing capabilities continuously.
By leveraging these tools, organizations can detect anomalies, prevent system failures, and ensure data integrity—all essential for maintaining trust in automated systems that operate in real-time. Moreover, as industries continue to evolve with the advent of IoT, AI, and machine learning, the need for adaptable, scalable, and resilient testing strategies will only grow.
Ultimately, investing in real-time data testing not only enhances operational reliability but also positions organizations to respond swiftly to changing conditions, innovate faster, and maintain a competitive edge in their respective markets. As technology continues to advance, embracing real-time testing will be vital for organizations aiming to harness the full potential of their data-driven applications.