Artificial Intelligence (AI) is revolutionizing industries worldwide, offering unprecedented opportunities for innovation. However, alongside its transformative potential, there is a critical need to address ethical concerns in AI development and deployment.
Ethical AI testing ensures that AI systems not only perform effectively but also uphold ethical standards and societal values.
As AI becomes integral to decision-making processes, issues like algorithmic bias, transparency, data privacy, and accountability are increasingly vital. Balancing innovation with responsibility is crucial to building trust and ensuring AI’s positive impact on society.
This article explores the complexities of ethical AI testing, examining principles, challenges, strategies, and real-world examples to underscore the importance of ethical considerations in AI advancement.
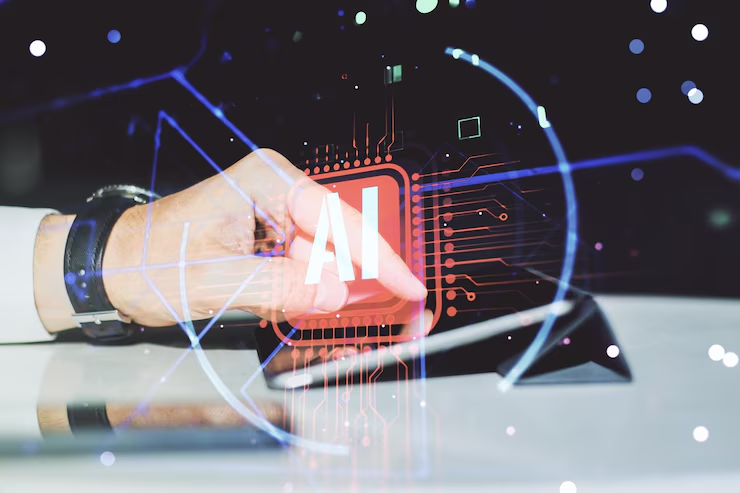
Understanding Ethical AI Testing
Ethical AI testing is essential to ensure that artificial intelligence systems are developed and deployed in a manner that aligns with moral principles and societal values. It encompasses a set of practices and principles aimed at addressing potential biases, ensuring transparency, protecting data privacy, and establishing accountability within AI systems.
Definition of Ethical AI Testing
Ethical AI testing involves evaluating AI systems not only for their technical performance but also for their ethical implications. It seeks to identify and mitigate biases that could lead to discriminatory outcomes, ensure transparency in how AI decisions are made, safeguard individuals’ privacy rights, and establish mechanisms for accountability when AI systems make decisions or recommendations.
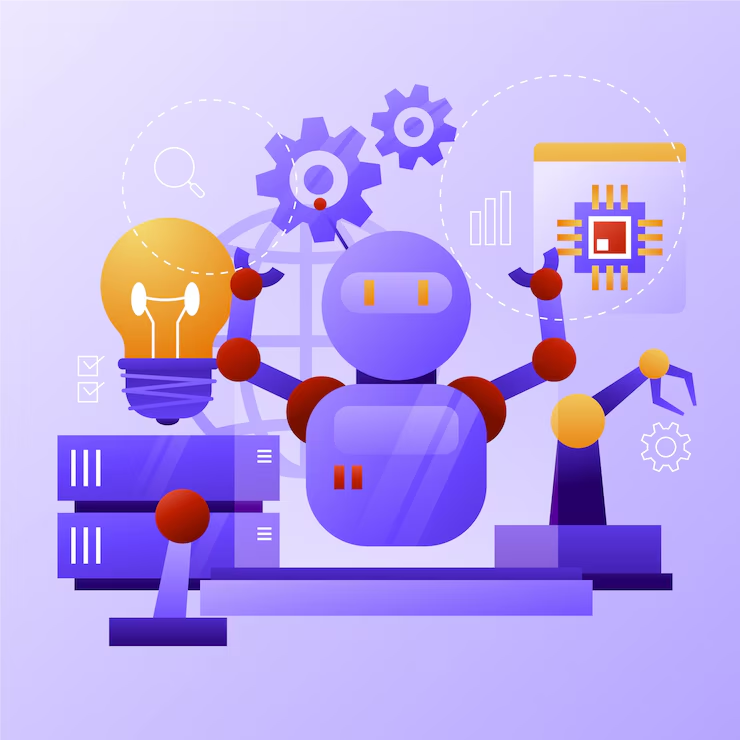
Key Ethical Principles in AI Development
Several key ethical principles guide the practice of ethical AI testing:
- Fairness and Bias Mitigation: Ensuring AI systems do not favor or discriminate against individuals or groups unfairly.
- Transparency and Explainability: Making AI decision-making processes understandable and providing explanations for outcomes to users and stakeholders.
- Privacy and Data Protection: Safeguarding sensitive information and respecting individuals’ rights to control their personal data.
- Accountability: Establishing mechanisms to assign responsibility for AI system behavior and outcomes, including recourse for errors or misuse.
Examples of Ethical Dilemmas in AI Testing
Ethical dilemmas in AI testing can arise from various scenarios, such as:
- Algorithmic Bias: AI systems that inadvertently discriminate against certain demographics due to biased training data.
- Privacy Concerns: AI applications that collect and use personal data without informed consent or appropriate safeguards.
- Lack of Transparency: Users are unable to understand why AI systems made specific decisions or recommendations, leading to mistrust.
The Importance of Ethical AI Testing
Ethical AI testing is crucial because it helps mitigate these risks and ensures that AI technologies are developed and deployed responsibly. By adhering to ethical principles, organizations can build trust with users, regulators, and the public, fostering broader acceptance and adoption of AI innovations.
Strategies for Ensuring Ethical AI Testing
Ensuring ethical AI testing involves implementing robust strategies to address key ethical considerations throughout the development and deployment lifecycle of AI systems. These strategies are essential for promoting fairness, transparency, privacy, and accountability. Here are some effective approaches:
1. Fairness and Bias Mitigation
To promote fairness and mitigate bias in AI systems, consider the following strategies:
- Diverse and Representative Data: Use diverse datasets that accurately represent the demographics and characteristics of the population the AI system will interact with.
- Bias Detection and Correction: Implement techniques to detect and mitigate biases during data collection, preprocessing, and algorithm development stages.
- Continuous Monitoring: Regularly monitor AI systems in production to detect and address biases that may emerge over time.
2. Transparency and Explainability
Enhancing transparency and explainability helps users understand AI system decisions and promotes trust. Strategies include:
- Interpretable Models: Use models that are interpretable and provide explanations for their decisions in a clear and understandable manner.
- Documentation: Document AI system design, data sources, and decision-making processes to enable external auditing and review.
- User-Friendly Interfaces: Design interfaces that communicate AI system capabilities, limitations, and potential biases to users effectively.
3. Privacy and Data Protection
Protecting privacy and ensuring data security are critical in ethical AI testing. Consider these strategies:
- Data Minimization: Collect and store only the necessary data required for the AI system’s function, minimizing the risk of unauthorized access or misuse.
- Anonymization and Encryption: Apply techniques such as anonymization and encryption to protect sensitive data throughout its lifecycle.
- Compliance with Regulations: Ensure AI systems comply with relevant data protection regulations and industry standards.
4. Accountability
Establishing accountability mechanisms helps mitigate risks associated with AI decision-making. Strategies include:
- Traceability: Maintain records of AI system operations, including data inputs, model decisions, and outcomes, to trace errors or biases.
- Ethics Committees: Form interdisciplinary ethics committees to review AI projects, assess potential ethical impacts, and provide guidance on responsible AI development.
- Clear Policies and Procedures: Define clear policies and procedures for handling ethical issues, including mechanisms for addressing user complaints or ethical concerns.
5. Stakeholder Engagement and Education
Engaging stakeholders and educating them about ethical AI principles foster a shared understanding and commitment to ethical practices. Strategies include:
- Training Programs: Provide training on ethical AI principles and best practices for developers, data scientists, and other stakeholders involved in AI projects.
- Consultation with Ethical Experts: Seek input from ethicists, social scientists, and other experts to anticipate and address ethical challenges.
- Public Dialogue: Foster open dialogue with users, communities, and policymakers to solicit feedback and address concerns about AI technologies.
Balancing Innovation with Responsibility
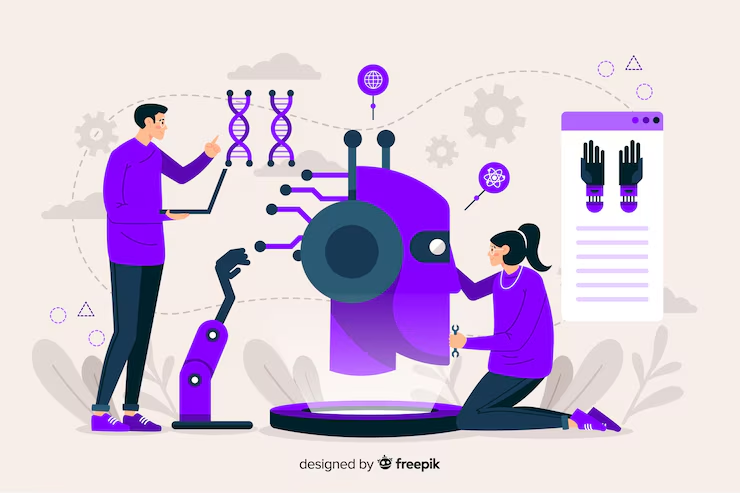
In the realm of artificial intelligence (AI), balancing innovation with responsibility is crucial to harnessing its full potential while mitigating ethical risks and societal concerns. This balance requires careful consideration of the implications of AI technologies on individuals, communities, and broader society. Here are key aspects to consider:
Ethical Considerations
AI innovation must be guided by ethical principles to ensure it benefits society as a whole. Key ethical considerations include:
- Fairness and Bias: Ensuring AI systems do not discriminate or perpetuate biases that could harm marginalized groups.
- Transparency: Making AI systems transparent so users understand how decisions are made and can trust the technology.
- Privacy: Protecting user data and respecting privacy rights throughout the AI lifecycle.
- Accountability: Establishing mechanisms to hold AI developers and deployers accountable for the outcomes of AI systems.
Responsible Development Practices
To balance innovation with responsibility, organizations should adopt responsible development practices:
- Ethics by Design: Integrate ethical considerations into the design phase of AI projects to proactively address potential risks.
- Risk Assessment: Conduct thorough risk assessments to identify and mitigate ethical, social, and legal risks associated with AI deployment.
- Continuous Monitoring: Regularly monitor AI systems in operation to detect and address ethical issues that may arise over time.
- Engagement with Stakeholders: Seek input from diverse stakeholders, including experts, users, and affected communities, to understand their concerns and perspectives.
Regulatory and Policy Frameworks
Governments and regulatory bodies play a crucial role in establishing frameworks that promote responsible AI innovation:
- Ethical Guidelines: Develop and enforce ethical guidelines and standards for AI development, deployment, and use.
- Compliance: Ensure AI systems comply with existing laws and regulations related to privacy, data protection, discrimination, and consumer rights.
- Incentives for Responsible AI: Provide incentives, such as funding and regulatory relief, for organizations that prioritize responsible AI practices.
Public Awareness and Education
Educating the public about AI technologies and their implications is essential for fostering informed discussions and decisions:
- Public Dialogue: Foster open and inclusive discussions about AI’s potential benefits and risks with the general public, policymakers, and civil society organizations.
- Education Programs: Develop educational programs to raise awareness about AI ethics, helping individuals make informed choices about AI-enabled products and services.
- Media and Communication: Ensure accurate and balanced reporting on AI developments to avoid misinformation and promote understanding.
Collaboration and International Cooperation
Given the global nature of AI technologies, collaboration and cooperation among stakeholders at national and international levels are essential:
- International Standards: Collaborate with other countries and international organizations to develop harmonized standards and norms for ethical AI.
- Information Sharing: Share best practices, research findings, and lessons learned in ethical AI testing and deployment across borders.
- Global Governance: Work towards establishing global governance frameworks that promote ethical AI practices and address cross-border challenges.
Conclusion
Achieving a balance between innovation and responsibility in artificial intelligence (AI) development is crucial for maximizing its benefits while safeguarding ethical standards and societal values. Throughout this discussion, we have highlighted key strategies such as ethical AI testing, responsible development practices, regulatory frameworks, stakeholder engagement, and public awareness.
Ethical AI testing ensures fairness, transparency, privacy protection, and accountability in AI systems. By embedding ethical considerations from the outset and continuously monitoring AI deployments, organizations can mitigate risks and build trust among users and stakeholders.
Responsible development practices, coupled with clear regulatory frameworks and international collaboration, are essential for setting standards that promote ethical AI globally. Educating the public about AI’s capabilities and ethical implications fosters informed discussions and decisions.
In conclusion, embracing innovation with a commitment to ethical principles allows us to harness AI’s potential for societal good. By advancing AI technologies responsibly, we can create a future where innovation enhances human well-being while respecting ethical boundaries and promoting trust in AI systems.