In the rapidly evolving world of telecommunications, wireless networks play a pivotal role in ensuring seamless connectivity and data transmission across the globe.
As the demand for faster, more reliable, and efficient wireless networks grows, the integration of artificial intelligence (AI) into these networks has become increasingly crucial.
AI, with its ability to analyze vast amounts of data and make real-time decisions, is revolutionizing the way wireless networks operate, optimizing their performance and enhancing overall efficiency.
Artificial intelligence encompasses a range of technologies, including machine learning, neural networks, and advanced algorithms, that enable machines to perform tasks typically requiring human intelligence.
In the context of wireless networks, AI can be employed to manage network traffic, allocate resources dynamically, mitigate interference, and even predict maintenance needs.
This integration not only improves the user experience by providing faster and more reliable connections but also reduces operational costs and enhances energy efficiency.
The importance of optimizing performance and efficiency in wireless networks cannot be overstated.
As we move towards an increasingly connected world with the proliferation of Internet of Things (IoT) devices, smart cities, and autonomous vehicles, the demand for robust and efficient wireless networks will continue to escalate.
AI offers a promising solution to meet these demands, driving innovation and enabling the development of next-generation wireless technologies.
In this article, we will explore the integration of AI in wireless networks, examining its role in enhancing performance and boosting efficiency.
We will delve into key components and technologies, discuss real-world applications and benefits, address challenges and ethical considerations, and look ahead to future trends and innovations in this exciting field.
By understanding the impact of AI on wireless networks, we can better appreciate its potential to transform the way we communicate and interact in our increasingly digital world.
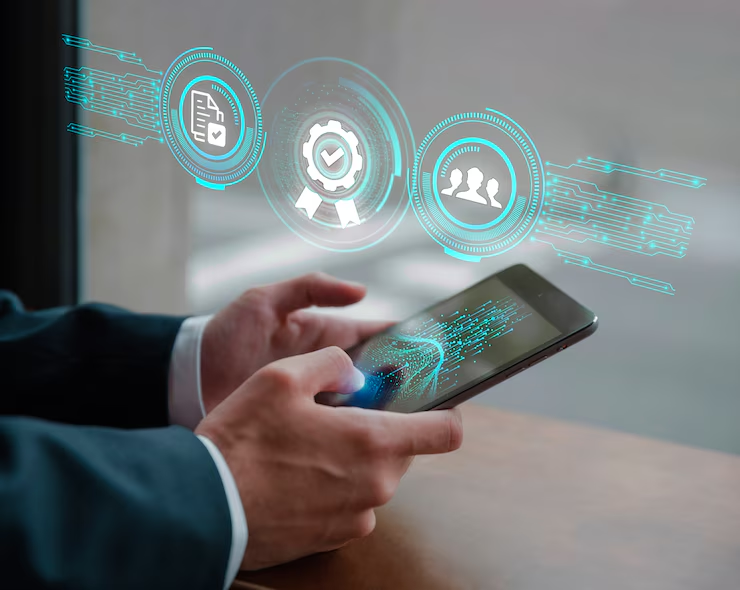
The Integration of AI in Wireless Networks
Historical Context and Evolution
The integration of artificial intelligence (AI) into wireless networks marks a significant milestone in the evolution of telecommunications.
Historically, wireless networks have relied on traditional, rule-based systems to manage and optimize their operations.
While these systems were effective to a certain extent, they often fell short in handling the increasing complexity and dynamic nature of modern wireless environments.
The advent of AI has brought about a paradigm shift, offering sophisticated tools and techniques to enhance network performance and efficiency.
The journey of AI integration in wireless networks began with the development of basic machine learning algorithms capable of analyzing network traffic patterns and making rudimentary adjustments.
Over time, advancements in AI research have led to the creation of more sophisticated models, such as deep learning and reinforcement learning, which can handle vast amounts of data and make real-time decisions.
These technologies have paved the way for more intelligent and adaptive wireless networks, capable of self-optimization and self-healing.
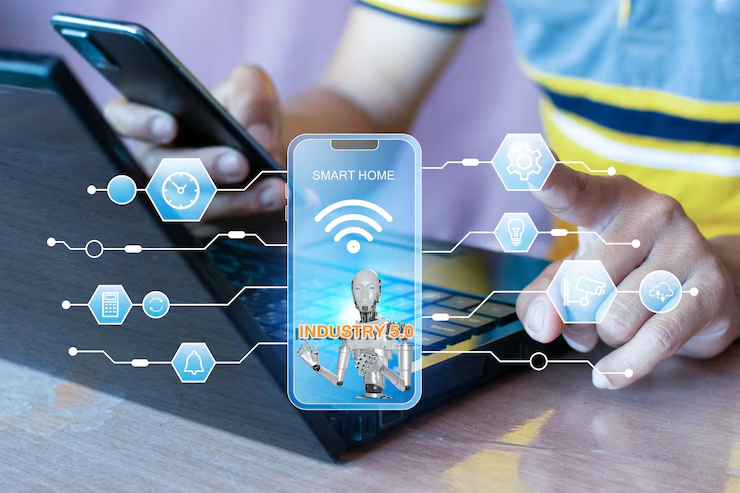
Key Components and Technologies
The successful integration of AI into wireless networks relies on several key components and technologies.
At the core of this integration are AI algorithms and machine learning models that process and analyze network data to optimize various aspects of network performance.
These models are trained on large datasets, enabling them to identify patterns and predict future network conditions with high accuracy.
One of the fundamental technologies driving AI in wireless networks is deep learning, a subset of machine learning that uses neural networks with multiple layers to analyze complex data.
Deep learning models excel in recognizing patterns and making predictions, making them ideal for tasks such as network traffic prediction, interference detection, and resource allocation.
Reinforcement learning, another crucial technology, allows AI systems to learn and adapt through trial and error.
In wireless networks, reinforcement learning can be used to develop algorithms that dynamically adjust network parameters to optimize performance based on real-time feedback.
This adaptive capability is particularly valuable in managing the ever-changing conditions of wireless environments.
In addition to algorithms and models, the integration of AI in wireless networks also requires robust hardware and software infrastructure.
This includes high-performance computing resources, such as graphics processing units (GPUs) and tensor processing units (TPUs), which are essential for training and running AI models.
Moreover, specialized software platforms and frameworks, like TensorFlow and PyTorch, provide the tools needed to develop and deploy AI solutions in wireless networks.
Enhancing Performance with AI
Network Traffic Management
Artificial intelligence (AI) plays a crucial role in enhancing the performance of wireless networks through effective network traffic management.
Traditional approaches to traffic management often rely on static rules and thresholds, which can be limited in their ability to adapt to dynamic network conditions and fluctuations in user demand.
AI-powered solutions, on the other hand, leverage advanced machine learning algorithms to analyze historical data, predict future traffic patterns, and make proactive adjustments in real time.
AI-driven Traffic Prediction: One of the primary benefits of AI in network traffic management is its ability to accurately predict traffic patterns based on historical data and current network conditions.
By analyzing data such as user behavior, device types, and application usage, AI algorithms can forecast peak periods of network usage and allocate resources accordingly.
This predictive capability allows wireless networks to preemptively adjust bandwidth allocation and routing paths to prevent congestion and ensure optimal performance for users.
Dynamic Traffic Routing: AI enables dynamic traffic routing, where network traffic is intelligently distributed across available paths based on current conditions.
This dynamic routing helps in load balancing, minimizing latency, and improving overall network efficiency.
For example, AI algorithms can reroute traffic away from congested areas or unreliable links, ensuring consistent connectivity and reducing packet loss.
Case Studies and Benefits: Real-world implementations of AI-driven traffic management have demonstrated significant improvements in network performance and user experience.
Telecom operators and network providers have reported reduced latency, increased throughput, and enhanced reliability by implementing AI-powered traffic management solutions.
These improvements translate into better service quality for users, particularly in high-density urban areas or during peak usage periods.
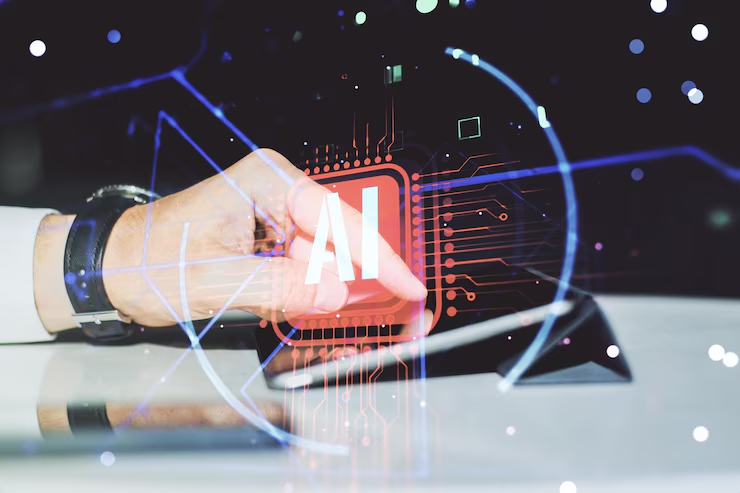
Boosting Efficiency through AI
Energy Efficiency
Artificial intelligence (AI) is revolutionizing energy efficiency in wireless networks by optimizing resource usage and reducing power consumption without compromising performance.
Traditional network infrastructures often operate at fixed power levels, regardless of fluctuating demand or environmental conditions.
AI-powered solutions, however, enable adaptive and intelligent management of network resources, resulting in significant energy savings and sustainability benefits.
AI-driven Resource Management
AI algorithms analyze real-time data on network traffic, user behavior, and environmental factors to dynamically adjust power levels and optimize energy consumption.
By identifying periods of low demand or network inactivity, AI can intelligently scale down power consumption in specific network components, such as base stations and routers, while maintaining service quality.
This adaptive approach not only reduces operational costs but also minimizes carbon footprint, contributing to a greener and more sustainable network infrastructure.
Case Studies and Benefits: Deployments of AI-driven energy management systems have shown substantial improvements in energy efficiency across various wireless networks.
Mobile operators and infrastructure providers have reported significant reductions in energy consumption and operational costs, while simultaneously enhancing network reliability and uptime.
For instance, AI algorithms have been instrumental in powering down inactive network components during off-peak hours, leading to measurable energy savings without compromising network readiness.
Predictive Maintenance
AI-powered predictive maintenance is another key area where efficiency gains are realized in wireless networks.
Traditional maintenance practices often rely on scheduled inspections and reactive repairs, which can be costly and disruptive to network operations.
In contrast, AI-enabled predictive maintenance uses machine learning algorithms to analyze historical performance data, identify potential equipment failures before they occur, and schedule maintenance proactively.
Proactive Fault Detection: AI algorithms continuously monitor network performance metrics, such as signal strength, equipment temperature, and error rates, to detect anomalies and early warning signs of potential failures.
By leveraging predictive analytics, network operators can address emerging issues promptly, replace faulty components proactively, and minimize downtime.
This proactive approach not only improves network reliability but also reduces operational costs associated with unplanned maintenance and repairs.
Implementation and Impact: Real-world implementations of AI-driven predictive maintenance have demonstrated tangible benefits across wireless networks.
Telecom operators have reported improved network availability, reduced maintenance costs, and enhanced user satisfaction by adopting AI-based predictive maintenance solutions.
By optimizing maintenance schedules and maximizing equipment lifespan, AI helps achieve operational efficiencies and ensure seamless service delivery.
Conclusion
Artificial intelligence (AI) has emerged as a transformative force in the realm of wireless networks, revolutionizing how networks are managed, optimized, and maintained.
Through advanced machine learning algorithms and predictive analytics, AI enables wireless networks to achieve unprecedented levels of performance, efficiency, and reliability.
AI’s impact on wireless networks is profound. In network traffic management, AI-driven solutions predict and manage traffic patterns in real time, optimizing bandwidth allocation and routing paths to ensure seamless connectivity and minimal latency.
This proactive approach enhances user experience and supports the growing demand for high-speed data transmission.
Moreover, AI plays a crucial role in boosting efficiency by optimizing energy consumption and reducing operational costs.
AI-powered resource management dynamically adjusts power levels based on network demand and environmental conditions, leading to significant energy savings and contributing to sustainable network operations.
Additionally, predictive maintenance powered by AI minimizes downtime by identifying and addressing potential equipment failures before they impact network performance, thereby enhancing reliability and reducing maintenance costs.
Looking ahead, the integration of AI in wireless networks holds promise for continued innovation and improvement.
Future advancements in AI technologies, such as quantum computing and enhanced machine learning models, will further enhance the capabilities of wireless networks, enabling them to meet the evolving demands of digital connectivity and emerging technologies.
In conclusion, AI’s role in wireless networks represents a paradigm shift towards smarter, more adaptive network management strategies.
By harnessing the power of AI, wireless networks are poised to deliver enhanced performance, greater efficiency, and superior user experiences, shaping the future of telecommunications in our interconnected world.