In today’s digital era, Digital Twin technology has emerged as a game-changer across various industries, enabling organizations to create virtual replicas of physical assets, systems, and processes. By mirroring real-world conditions, digital twins facilitate better decision-making, predictive analytics, and performance optimization. However, as industries generate vast amounts of data, Artificial Intelligence (AI) is playing a crucial role in enhancing the capabilities of digital twins.
AI-powered digital twins go beyond static modeling by enabling real-time simulations, adaptive learning, and automated decision-making. AI enhances the accuracy of predictions, optimizes operations, and improves the efficiency of simulations by integrating machine learning (ML), deep learning, and advanced data analytics. As a result, industries such as manufacturing, aerospace, healthcare, smart cities, and energy are leveraging AI-driven digital twins to revolutionize their workflows and drive innovation.
This article explores the role of AI in digital twin technology, highlighting its impact on simulations, predictive analytics, and real-time decision-making. It also examines key industry applications, challenges, and the future trends shaping the evolution of AI-powered digital twins.
The Role of AI in Digital Twin Technology
The integration of Artificial Intelligence (AI) with Digital Twin technology has significantly enhanced the capabilities of virtual models, making them more dynamic, adaptive, and intelligent. Traditional digital twins rely on historical data and sensor inputs to simulate real-world conditions, but AI takes this further by enabling predictive analytics, real-time decision-making, and automated optimizations.
Here’s how AI is transforming digital twin technology:
1. Enhancing Data Processing and Real-Time Analytics
AI enables digital twins to process vast amounts of real-time data from IoT sensors, cloud systems, and enterprise applications. By leveraging machine learning (ML) and deep learning models, AI-driven digital twins can identify patterns, detect anomalies, and provide insights faster and more accurately than traditional methods.
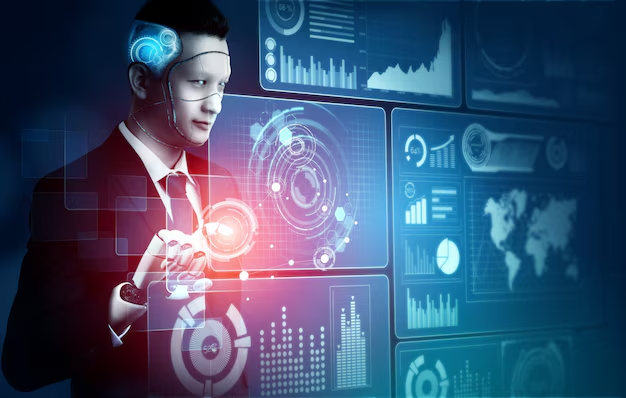
2. AI-Driven Predictive Maintenance and Fault Detection
One of the most impactful applications of AI in digital twins is predictive maintenance. AI algorithms analyze sensor data to predict equipment failures before they occur, reducing downtime and optimizing maintenance schedules. This is widely used in aerospace, manufacturing, and energy industries, where system failures can be costly and even hazardous.
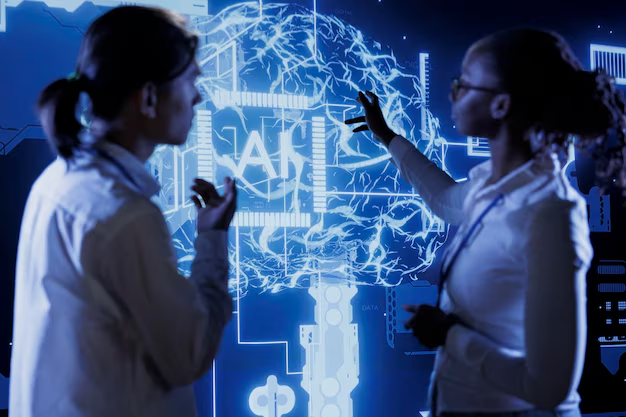
3. Machine Learning Models for Behavioral Predictions
Digital twins use AI-powered behavioral modeling to simulate different scenarios and predict future states of a system. ML models continuously learn from historical data and operational trends, allowing digital twins to make proactive recommendations for optimizing production lines, logistics, and infrastructure management.
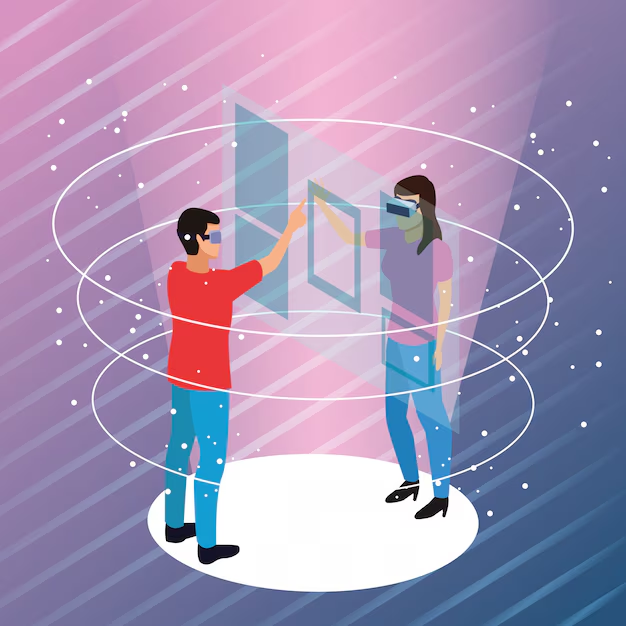
4. AI for Automated Decision-Making in Simulations
AI enhances digital twins by automating complex decision-making processes. For example:
- In smart factories, AI-driven digital twins can autonomously adjust production workflows to optimize efficiency.
- In healthcare, AI-powered patient digital twins can assist doctors in making personalized treatment recommendations based on simulated responses to medications.
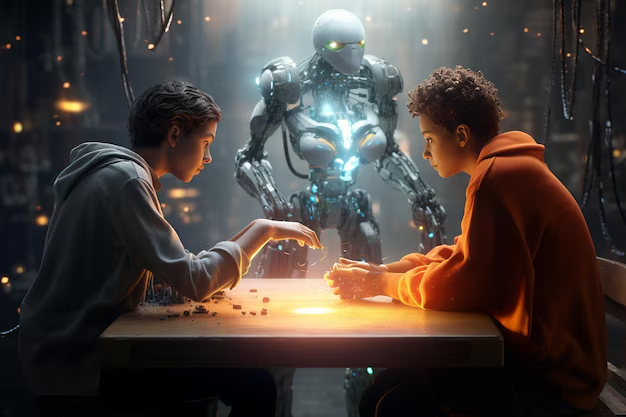
5. Adaptive Learning and Self-Optimization
AI enables digital twins to continuously evolve by learning from real-world data. With reinforcement learning, digital twins can self-optimize and improve over time, refining their models based on real-world feedback. This is crucial for applications such as autonomous vehicles, industrial automation, and smart grids
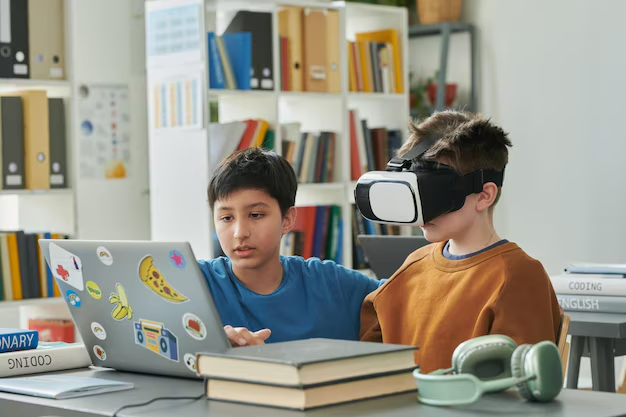
AI-Powered Simulations and Modeling
Artificial Intelligence (AI) is revolutionizing simulations and modeling within digital twin technology, making them more accurate, efficient, and adaptive. Traditional simulations rely on predefined mathematical models and historical data, but AI enhances these by enabling real-time learning, predictive modeling, and generative design. AI-driven digital twins can simulate complex, multi-domain interactions, allowing businesses to test scenarios, optimize processes, and improve decision-making.
1. How AI Improves Simulation Accuracy and Efficiency
AI enhances simulations by:
- Reducing computational load using optimized ML models instead of brute-force calculations.
- Improving prediction accuracy by dynamically adjusting models based on real-time feedback.
- Handling uncertainties through probabilistic AI models, making simulations more robust.
For instance, in aerospace engineering, AI-driven digital twins simulate aircraft performance under varied weather and operational conditions, reducing the need for extensive physical testing.
2. Generative AI for Creating Synthetic Data for Testing
Generative AI enables digital twins to create synthetic datasets for training and validation. This is useful when real-world data is scarce, expensive, or sensitive.
- In healthcare, AI generates patient-specific digital twins to test personalized treatment plans.
- In manufacturing, AI-driven twins simulate different design iterations before physical prototyping.
3. AI in Multi-Physics and Multi-Domain Simulations
Real-world systems involve multiple interacting forces (e.g., thermal, mechanical, electrical, and fluid dynamics). AI enhances digital twins by:
- Integrating data from multiple domains for comprehensive simulations.
- Enabling cross-disciplinary optimization, such as aerodynamic and structural optimizations in automotive design.
For example, in energy grid management, AI-powered digital twins simulate power fluctuations, demand response, and renewable energy integration to ensure stability.
4. Reinforcement Learning for Optimizing Digital Twin Performance
Reinforcement learning (RL) allows digital twins to self-optimize by learning from past interactions.
- In autonomous systems, RL-driven digital twins improve robot navigation and process automation.
- In smart cities, AI-based digital twins optimize traffic flow by simulating and adjusting real-time traffic conditions.
Key Industry Applications of AI-Enhanced Digital Twins
AI-powered digital twins are transforming multiple industries by enabling real-time monitoring, predictive analytics, and autonomous decision-making. By combining AI, IoT, and simulation models, organizations can optimize performance, reduce costs, and improve operational efficiency. Here are some key industries leveraging AI-enhanced digital twins:
1. Manufacturing: Smart Factories and Process Optimization
- AI-driven digital twins monitor production lines, predicting equipment failures and optimizing workflows.
- Machine learning models analyze sensor data to reduce downtime and improve product quality.
- Example: Siemens uses AI-powered digital twins to improve factory efficiency and reduce production defects.
2. Aerospace & Defense: Aircraft Performance Simulation and Predictive Maintenance
- AI-enhanced digital twins simulate aircraft behavior under different conditions, reducing the need for extensive physical testing.
- Predictive maintenance helps identify potential failures in engines and avionics before they occur.
- Example: Boeing and Airbus use AI-powered digital twins to optimize aircraft performance and enhance safety measures.
3. Healthcare: AI-Based Patient Digital Twins for Personalized Medicine
- AI-powered digital twins model human physiology to test the effects of medications and treatments before actual application.
- Personalized treatment plans are developed based on real-time patient data and AI simulations.
- Example: AI-driven digital twins are used in cardiology to predict heart disease progression and assist in surgical planning.
4. Smart Cities: AI-Driven Urban Planning and Traffic Management
- Digital twins of cities help simulate traffic congestion, energy consumption, and infrastructure changes.
- AI optimizes traffic signals and routes to reduce carbon emissions and travel time.
- Example: Singapore uses AI-powered digital twins for real-time urban planning and smart traffic management.
5. Energy & Utilities: Power Grid Optimization and Renewable Energy Management
- AI-driven digital twins simulate power demand fluctuations, optimize grid stability, and predict equipment failures.
- AI integrates renewable energy sources like solar and wind into smart grids for better energy efficiency.
- Example: GE Digital uses AI-enhanced digital twins to improve power plant operations and asset management.
6. Automotive: AI in Autonomous Vehicles and Smart Manufacturing
- AI-powered digital twins simulate self-driving car scenarios, improving safety and efficiency.
- Smart manufacturing digital twins optimize vehicle production, battery performance, and supply chains.
- Example: Tesla uses AI-based digital twins to enhance autonomous driving systems and EV performance.
7. Telecommunications: AI for Network Optimization and 5G Deployment
- AI-driven digital twins simulate network traffic, signal interference, and infrastructure planning.
- Predictive analytics optimize 5G deployment and bandwidth allocation.
- Example: Telecom providers use AI-powered twins to optimize cell tower placement and signal strength.
Conclusion
AI-powered digital twins are transforming industries by enabling real-time insights, predictive analytics, and autonomous decision-making. From smart factories and healthcare to aerospace and smart cities, these intelligent models are revolutionizing the way businesses simulate, monitor, and optimize their operations. By integrating machine learning, IoT, and advanced simulations, AI-driven digital twins provide unprecedented accuracy, efficiency, and adaptability.
However, challenges such as data security, computational complexity, and integration with legacy systems must be addressed for widespread adoption. As AI technology continues to evolve, digital twins will become even more sophisticated, self-learning, and integral to the digital transformation of industries.
Ultimately, AI-enhanced digital twins are not just a technological advancement—they are a strategic necessity for organizations aiming to stay ahead in a rapidly evolving world. Investing in this technology today will pave the way for smarter decision-making, operational efficiency, and long-term innovation.